Deploying speech recognition locally versus the cloud
December 17, 2015
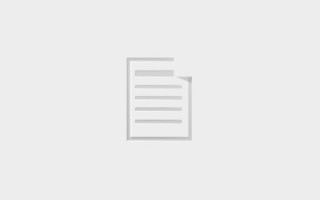
Everyone familiar with Siri, Google Now, Cortana, S-Voice, and/or Echo is familiar with the progress and improvement in speech recognition over the pa...
Everyone familiar with Siri, Google Now, Cortana, S-Voice, and/or Echo is familiar with the progress and improvement in speech recognition over the past decade. Much of this improvement comes from cloud-based recognizers deploying “deep learning” on big data.
Although it’s often out of the spotlight, there’s been lots of progress in speech recognition for embedded systems. In fact, most of the major speech engines deploy a combination of embedded plus cloud-based recognition. This is most noticeable in commands like “Hey Siri,” “OK Google,” “Hey Cortana,” “Hi Galaxy,” and “Alexa.” All of these cloud-based recognition systems use embedded “trigger” phrases to open the cloud connection to ready itself for the speech recognition.
Embedded trigger phrases allow a few improvements and practicalities over cloud-based approaches. For one, having an embedded recognizer “always on” is a lot less creepy than having your conversations going up to the clouds for Google and others to analyze any way they want. Since it’s on-device, there’s no speech recording or transmitting until the trigger phrase is spoken, and the trigger listening is done in real time without your speech being sent off.
There are also practical reasons for an embedded wake-up trigger, and a leading one is power consumption. Running exclusively on in the cloud would require lots of data transfer and analysis, making a battery-operated or “green” product impractical. Many major DSP companies have solutions for “always on” DSPs that run Sensory’s TrulyHandsfree wake-up trigger options at 2 mA or less. With sound activity detection schemes, the average battery drain can be under 1 mA, placing it in the realm of battery leakage.
Other popular uses of embedded speech recognition are in devices that want fast and accurate responses to limited commands. One of my favorite examples is in the Samsung Galaxy smartphones where, in camera mode, users can enable voice commands to take pictures. This works for me from up to 20 feet away in a quiet setting or 5 feet in a noisier location. It’s an awesome alternative to carrying around a selfie stick, and whenever I show this feature to people they quickly get it and love it.
Embedded speaker verification is also being deployed more frequently and is often incorporated into a wake-up trigger to decrease the probability that others can wake up your device. With speech recognition and speaker verification, there’s always a trade-off between false accepts (accepting the wrong user) and false rejects (rejecting the right user). The preferred wake-up trigger setting is often to keep false rejects extremely low at the cost of occasionally letting the right person in. In systems requiring more sophisticated speaker verification for security, it’s possible to deploy more complex algorithms that don’t require the lowest power consumption, to gain better accuracy at the cost of increasing current consumption.
As consumer products and mobile phones use more sophisticated processors, I expect a higher percentage of speech recognition use will move to the embedded devices, and a “layered” speech-recognition approach will emerge, whereby a fast initial analysis is done on device and responded to if the device has a high confidence of success (self-perception), but passed to the cloud if it’s less sure of its response or if a cloud-based search is required.
Todd Mozer is the CEO of Sensory. He holds over a dozen patents in speech technology and has been involved in previous startups that reached IPO or were acquired by public companies. Todd holds an MBA from Stanford University, and has technical experience in machine learning, semiconductors, speech recognition, computer vision, and embedded software.