Using AI Techniques for Improved Medical Imaging
March 18, 2021
Blog
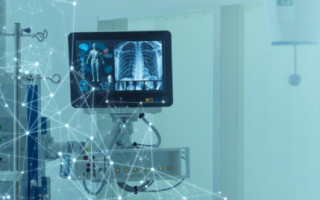
The analysts all agree—the spend for AI hardware (and software) will be going through the roof in short order.
I know we’re not breaking any new ground with that statement, but it needs to be said, to lay the foundation for the rest of this blog. Some spaces will see bigger increases than others, and one application in particular that’s poised for huge growth is imaging, especially in the medical arena.
Medical (sometimes referred to as healthcare) affects everyone, particularly because we have an aging population. The latest hardware brings a lot to the party in terms of performance to solve the needs of high responsiveness, substantially better accuracy, and improved imaging capabilities. The bottom line is that GPU computing has the ability to accelerate and improve medical imaging to help medical professionals make better and faster decisions.
Thanks to NVIDIA‘s advances in GPU technology, the industry is seeing an evolution in the way that medical images can be reconstructed. Taking that next step, actually implementing the NVIDIA technology, is where ADLINK Technology shines. In practice, that GPU can convert signals into 2D or 3D images, which requires a series of complex computations.
GPU solutions permit intelligent video analytics (IVA), which takes video data and turns it into actionable insights. For its part, ADLINK focuses on Edge computing. Accelerating that Edge computing with a GPU enhances image quality, accelerates image construction, and performs image analysis to help the medical professional treat patients. At the end of the day, ADLINK is making medical imaging more widely available in clinical practices and surgical procedures.
It’s clear that no single company holds all the IP to make a successful platform. The partnership between ADLINK and NVIDIA allows for scalability and future-proofing. The GPU—NVIDIA’s expertise—obviously plays a significant role in medical imaging, particularly with the company’s GPU architecture and its various software stacks. ADLINK’s embedded mobile PCI express modules (MXMs) and GPU computing platforms, powered by NVIDIA GPUs, offer computing acceleration for these enhanced imaging applications today and in the future.
The Challenges of Medical Imaging
One time-tested approach to customizing platforms for medical applications is through the use of modules. The modules can house any assortment of processors to fit the specific application. For the applications referenced here, ADLINK’s embedded MXM GPU modules have the ability to boost performance beyond what can be achieved by a conventional CPU, including image processing and analytics, compute acceleration, and AI.
The company’s latest MXM GPU modules are designed with the NVIDIA Turing architecture, which integrates CUDA cores, RT cores, and Tensor cores in one GPU. For example, the EGX-MXM-RTX3000 module features advanced NVIDIA Turing GPU technology in an MXM 3.1 Type B form factor which is only one-fourth the size of full-length PCI Express graphics (PEG) cards. With a total graphics power starting at 80 W, MXM GPU modules are suited for size-, weight-, and power-constrained, mission-critical medical imaging applications, such as C-arms and other mobile equipment.
Also keep in mind that, like industrial applications, the medical space is looking for longevity in its products. Two to five years is not unusual, and we often see where 10 years of support is required for a platform. Hence, ADLINK is designing these systems using NVIDIA’s embedded GPU solutions, giving customers the confidence that longer life support will be available.
RDMA To the Rescue
Finally, a feature that’s been implemented in the latest ADLINK systems is NVIDIA’s GPUDirect Remote DMA (RDMA). In medical imaging applications, GPUDirect RDMA gives external data sources direct access to the GPU’s memory. Without this feature, data would be copied into a CPU’s memory before reaching the GPU, needlessly increasing data transmission delay and latency.
A typical use case for RDMA is ultrasound. In most cases, ultrasound makes use of a front-end device like an FPGA to do the analog-to-digital conversion before the data reaches the GPU. That’s potentially a lot of communications back and forth between the FPGA and the GPU. With RDMA enabled, the bandwidth increase provides the necessary performance.
While the goal of better medical imaging is an obvious one, it’s far from easy to implement. That goal is simply to take the right action, with the right data, at the right place, at the right time. The key to achieving this goal is in integrating domain knowledge with platforms and building blocks, then tailor those pieces for individual end-user needs.
To hear more about ADLINK’s medical-imaging products and technologies, check out the talk delivered by Zane Tsai, the Director of ADLINK’s Platform Product Center as part of NVIDIA’s upcoming GTC.